Uncovering JAPA
Reading Plans With Machines for Improved Efficiency
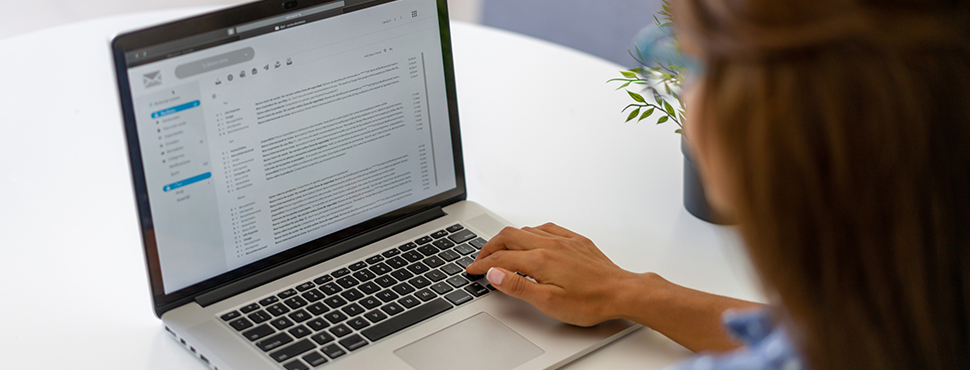
As the world encounters new and evolving challenges, planners must read up-to-date policies that inform practices. Yet, it seems impossible to read the planning documents from every municipality worldwide to understand how they approach these challenges.
Efficient Machine Coding for Planning Documents
How can planners be more efficient in staying informed on current issues? In "Using Natural Language Processing to Read Plans" (Journal of the American Planning Association, Vol. 89, No. 1), Xinyu Fu, Chaosu Li, and Wei Zhai propose an approach that uses a machine-based method to identify key takeaways from lengthy, technical documents quickly. However, is this process accurate? To answer this question of accuracy, they compared this machine-coding process to traditional hand-coding analyses.
Fu et al. analyzed 78 resiliency planning documents from the 100 Resilient Cities Network, totaling 7,788 pages or more than 14 million words. By focusing on resilience planning, the authors demonstrated how artificial intelligence, specifically natural language processing (NLP), can process several documents quickly.
NLP analyses could identify the main topics from an extensive collection of planning documents within minutes, at the expense of omitting some less-mentioned issues. In contrast, the traditional hand-coding analyses yielded more precise understandings at the cost of time and labor.
The authors distilled eight major planning topics from the sampled 78 documents:
- Infrastructure
- Economic development
- Water management
- Employment
- Urban governance
- Disaster management
- Urban development
- Housing
In particular, the authors noted that the top four topics made up more than half of the content in planning documents — meaning resilient planning documents seem biased towards infrastructural and economic development. However, the authors remind planners to consider the bias of the sampled sources — referencing that the Resilient Cities Network is a global network funded by the Rockefeller Foundation. The latter's motivations include promoting public-private partnership (and thus an emphasis on infrastructure and economic development).
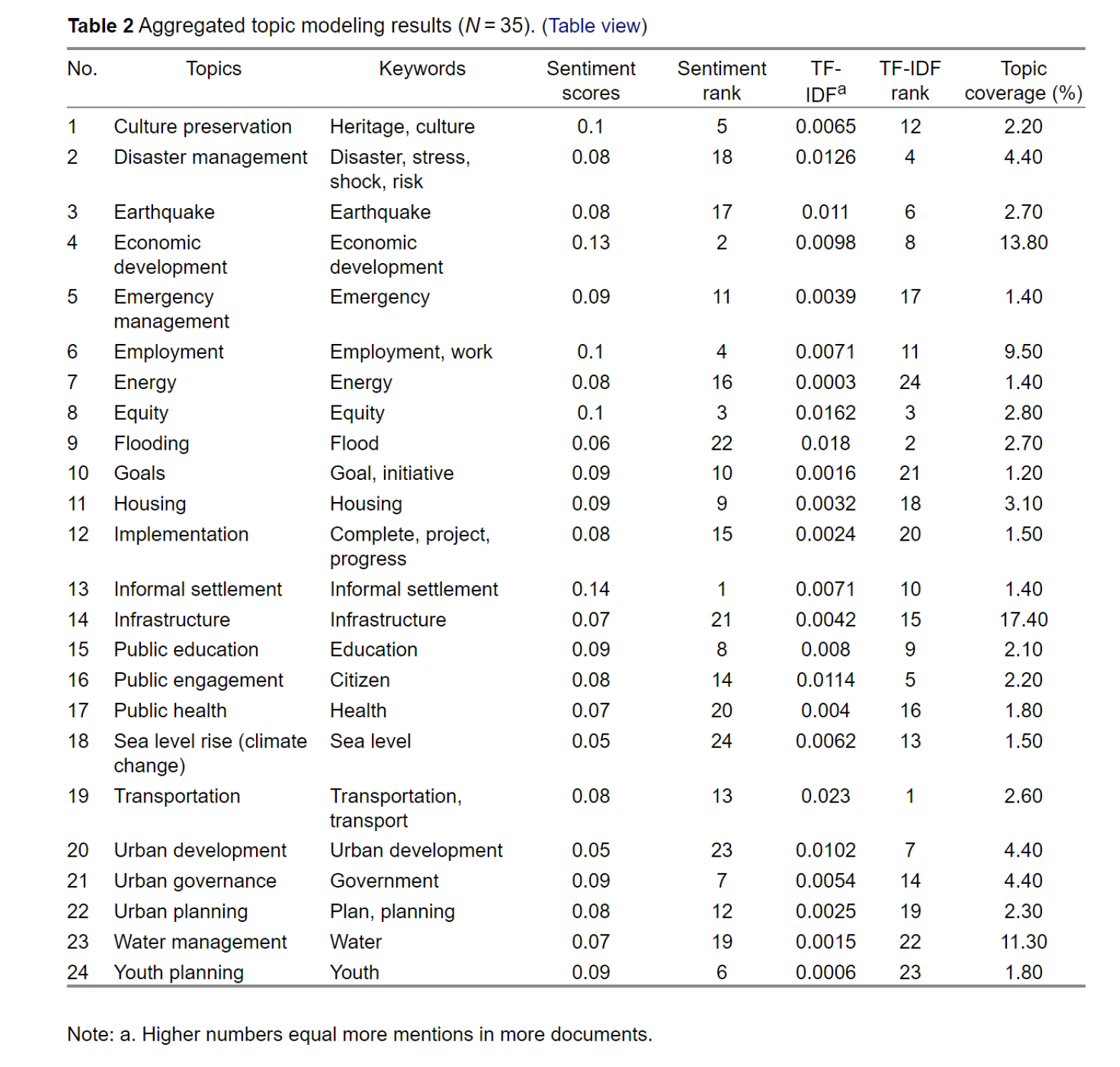
Table 2. Twenty-four key topics were distilled from the sampled planning documents.
Machine Learning Aids Planning Document Analysis
Fu et al.'s approach is an exciting challenge to use machine learning appropriately, specifically to assist with:
- Identifying Planning Priorities and Main Themes: Whereas it may have taken hours to distill the main themes from the planning documents studied, this method emphasizes the efficiency of NLP topic modeling compared to hand-coded, traditional content analysis. The authors recommend that planners who want to use topic modeling should use 10-20 topics for "short and simple plans" and 25 to 35 topics for longer, more complex plans.
- Using Machine-Identified Themes as a Roadmap of Further In-depth Reading: This study notes how NLP can identify specific plans that "have a higher coverage of one of the identified topics." This use of NLP narrows the sample to a few documents for planners to read more in-depth to distill specific planning strategies.
- Emphasizing the Importance of External Collaboration in Planning: The topics uncovered by the NLP process (such as "air quality and employment") signal a need for planners to coordinate with various agencies with more specific knowledge on these topics. As a result, the NLP process allows planners to have a working understanding of themes while identifying the sectors with whom planners should engage.
As a current planning student, I'm excited by the prospect of increasing the range and depth of documents I can reference because the NLP method can process more content, allowing a more comprehensive look at planning initiatives than otherwise permitted.
Top image: E+ - andreser