Uncovering JAPA
Can ChatGPT Analyze Public Feedback?
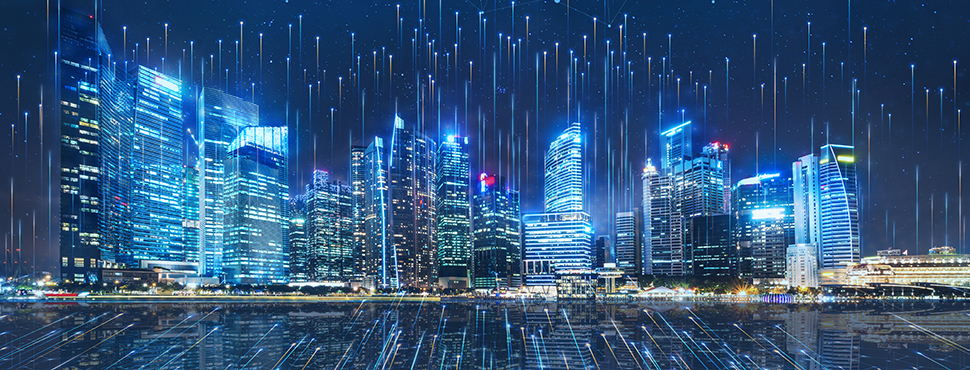
Online public engagement produces vast amounts of feedback data. Planners must efficiently derive insights from this massive dataset. Tools like ChatGPT have emerged as promising aids, mainstreaming artificial intelligence (AI) use and exciting planners about its potential in public engagement.
But do we know how ChatGPT compares to human analysis or other models' analyses? And what are the best ways to use this powerful tool?
In "Deciphering Public Voices in the Digital Era: Benchmarking ChatGPT for Analyzing Citizen Feedback in Hamilton, New Zealand" (Journal of the American Planning Association, Vol. 90, No. 3) Xinyu Fu, Thomas W. Sanchez, Chaosu Li, and Juliana Reu Junqueira explored how ChatGPT could be used to analyze an online public feedback dataset in response to a proposed local plan change.
This benchmark study used 2022 public feedback data from the Hamilton City Council's website. The council sought input on Plan Change 12, which would amend the district plan to align with new national housing development rules.
Comparing Prompts
The authors used zero-shot prompts with a large language model to generate summaries, identify topics, and perform sentiment analysis. Zero-shot prompting involves prompts without specific instructions or training data. This is the most widely used method. In contrast, few-shot prompting provides detailed instructions and training data with specific inputs and desired outputs.
The ChatGPT results were compared with those of human planners and two standard natural language processing techniques — latent Dirichlet allocation topic modeling and lexicon-based sentiment analysis.
Machine Effectiveness
Zero-shot prompts with ChatGPT effectively identify political stances (81.7% accuracy), reasons (87.3%), decisions sought (85.8%), and associated sentiments (94.1%). While the model has limitations, it shows promise for automating public feedback analysis, potentially saving time and costs. Few-shot prompting improves performance in complex tasks, like understanding planning jargon, where general-purpose models struggle without additional training.
These advancements in AI offer new opportunities for planners to analyze diverse community inputs efficiently. By leveraging AI's capabilities, planners can gain deeper insights into community needs and preferences, aiding more informed and equitable urban development strategies.
Genuine public engagement goes beyond collecting opinions; it involves understanding, synthesizing, and integrating viewpoints into actionable policies. This ensures community input shapes transparent and trusted decision-making.
The diverse challenges of public feedback — like language variation, subjective opinions, local context, and data limitations — highlight the need to establish robust practices for a local landmark before widespread adoption. This ensures its effectiveness in meeting community needs.
The authors urge users of large language models to fact-check results and always keep humans in the loop as the technology evolves.
Top image: Photo by iStock/Getty Images Plus