Planning Ethics and Generative AI
Translating principles of the AICP Code of Ethics into practical guidance for use of generative AI in planning
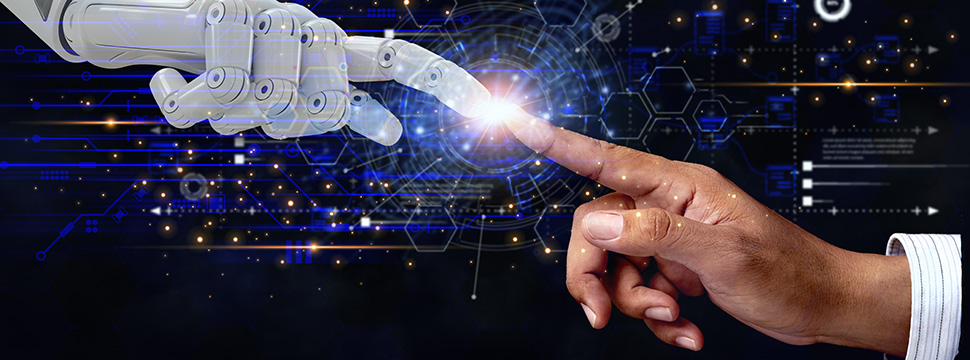
There have been increasing inquiries, adoption of policy, and progressive uptake associated with generative AI technology across the planning profession. The American Planning Association's Ethics Committee in particular has received a substantial volume of inquiries on the subject since as early as 2003.
Increasing AI Inquiries, Adoption in Planning
This blog, co-authored by the APA Technology Division's board and the APA Ethics Committee with input from planning practitioners and technologists, expands on the APA Technology Division's Open Letter reflecting on the changing landscape of planning practice as well as APA's ongoing efforts to support planners' strategic adoption of AI.
The following outlines four important ideas from the open letter to address concerns at the intersection of planning practice and the ethical use of artificial intelligence:
1. Be sensitive to THE bias and limitations of AI
Use it to improve judgment, but do not delegate wholesale authority to make decisions.
Relevant AICP Code of Ethics Sections: A.1.f., A.2.a.
Generative AI models like the ones used for text, image, and other media generation are trained on massive datasets pulled from the internet and other sources, which means they can absorb and perpetuate societal biases and skewed perspectives present in that training data. A possible silver lining is that the extent of bias in these models is auditable, and the severity of their biases should be benchmarked against the baseline bias observed in existing planning processes.
In accordance with Sections A.1.f. and A.2.a of the AICP Code of Ethics and Professional Conduct, to prevent the biases and limitations of generative AI from harming communities, including through the dissemination of misinformation and perpetuation of inequities and negative stereotypes, planners must develop an internal strategy and guidelines for uptake and use generative AI.
Planners must be prepared to guide and contextualize generated output through systematic and critical analysis toward compliance with ethical guidelines and organizational policies.
Example: Addressing Bias and Limitations of AI
Before the chatbot is made available to the public, John might:
- Share the issues he's encountered in the application with his supervisor and other project team members as soon as possible, communicating the potential harm that such issues might cause to its users.
- Work with the project team to revise the release timeline, project specifications, testing, and issue reporting options to ensure that appropriate controls are in place to prevent the sharing of inaccurate information with users.
2. Systematically check outputs
Disclose use whenever practicable and communicate capabilities and limitations.
Relevant AICP Code of Ethics Sections: A.2.a., B.1., B.14., B.16.
Since the large language models (LLMs) that fuel many of today's most popular generative AI tools are statistical models with limited fact-checking capabilities and contextual guardrails, they are also prone to error. Even the most advanced models at the time of this publication, OpenAI's GPT 4o, Anthropic's Claude 3.5 Sonnet, and Google's Gemini 1.5 Pro received accuracy scores ranging from 85.9 percent and 88.7 percent as of July 2024, indicating that, across a variety of tasks, these models still returned results that had between an 11.3 and 14.1 chance of being incorrect. It is not clear how these accuracy scores would translate to detailed planning tasks which are not the focus of these benchmarking exercises.
In accordance with Section A.2.a and Section B.1. of the AICP Code of Ethics, planners must provide timely, adequate, clear, accessible, and accurate information on planning issues to stakeholders, while AI can assist in summarizing such requirements but also poses risks of generating misleading or plagiarized outputs that planners must carefully verify and contextualize.
To prevent error and oversight stemming from inaccurate model outputs, planners must disclose the use and limitations of generative output and utilities and fill the role of "the human in the loop" to validate outputs.
Items 14 and 16 within Section B under "Quality and Integrity of Practice" note areas where the work of others should not be misrepresented or misattributed. Current models are notoriously regarded as 'black boxes' and LLM providers still struggle to ensure their products consistently and accurately provide attribution to sources presenting further challenges to their use in planning practice since there is not only a risk of inaccurate output, but also output missing attribution either to original sources or to the model itself.
Example: Verifying Outputs and Disclosing AI Use
Jane, a planner for a local government that is experiencing a surge in development applications but suffering from limited staff capacity, is using AI to assist with drafting staff reports for public hearings. Jane notices that the generated text for the staff reports often cites incorrect sections of code, misinterprets code requirements, or omits important information entirely.
Before submitting these reports for review to her director, Jane might:
- Review all generated text output for errors and omissions and manually revise the reports.
- Consult organizational requirements around disclosure of the use of generative AI technology and ensure attribution is given to all sources cited in the generated output.
3. Be proactive
Educate yourself about emergent technology and AI systems.
Relevant AICP Code of Ethics Sections: A.2.e., A.2.f., A.3.a., A.5.c.
In the United States, investment in AI reached $67.2 billion in 2023, according to Stanford University's 2024 AI Index Report. This growth underscores the need for AI literacy, emphasizing education and continued training both in academic planning programs and within planning practice to build awareness and core competencies.
Key to this is learning the risks and opportunities of using emergent technology systems such as AI and actions to best manage its ethical use. The NIST AI Risk Management Framework (AI RMF) and companion NIST AI RMF Playbook are comprehensive and evolving resources that can be applied to planning and to gain a broader understanding of how AI influences allied fields.
Equally important is ensuring good documentation in AI use and application, including understanding the limits of citation, which is essential for the transparency and responsible use of the technology. Utilizing guiding principles — such as the CLeAR (Comparable, Legible, Actionable, and Robust) Documentation Framework designed for the entire AI ecosystem of creators, users, evaluators, policymakers, and consumers — can help foster reliability and trust in planning outcomes moving forward.
Under Section A.2.e of the Code of Ethics, we have a unique responsibility to enhance our professional education and training in AI, ensuring we are equipped with the latest knowledge and skills to address the complex challenges of this modern force that influence the profession. This includes a commitment, as outlined in Section A.5.c, to obtaining ongoing professional upskilling throughout our careers, allowing us to remain current with evolving concepts such as AI technologies. This is also important for integrating the AI curriculum into planning degree programs and AICP CRM course content.
Furthermore, consistent with Section A.2.f, we must educate and empower the public about AI and its use in planning, fostering an informed and engaged community that can actively, and ethically, participate in planning processes.
In line with Section A.3.a, a critical aspect of our role is recognizing and eliminating historic patterns of inequity embedded in planning documents. By understanding these systemic issues and leveraging AI responsibly, we can avoid perpetuating digital biases.
Example: Upskill Yourself on AI
Avery, an environmental planner working with a state emergency management agency, is developing an AI model to enhance flood resilience by improving flood forecasts. Despite their hydrology expertise, Avery lacks formal training in AI, leading to challenges in data preprocessing, model selection, and training. Additionally, they fail to document data sources, preprocessing steps, and model parameters accurately. Consequently, the AI model sometimes produces inaccurate forecasts by referencing outdated data and omitting critical variables, posing risks to flood preparedness and response efforts.
Before sharing the results of the flood forecast at an internal workshop with the project team, Avery can:
- Conduct an analog review of the data and validate predictions against actual events to maintain accuracy and relevance, better understanding the risks and potential impacts of the AI model.
- Establish a protocol using peer-reviewed sources for detailed documentation to help track changes, identify errors, and provide a clear understanding of the model's development process, enhancing transparency and accuracy.
- Enroll in an online AI webinar or upskilling course to:
- Gain a deeper understanding of AI concepts, applications, and best practices in machine learning.
- Learn best practices for data preprocessing, model selection, training, and validation.
- Acquire proper documentation techniques, including citation protocols, to ensure transparency for future projects.
4. Do not disclose sensitive information
Regarding constituents or organizations using platforms that are not authorized within an organization.
Relevant AICP Code of Ethics Sections: A.4.b., B.13.
The sudden mainstream availability of generative AI utilities has introduced an abundance of benefits and threats to civil society. A recent study by Google found that generative AI misuse has enabled more effective interference from malicious actors including by enabling misinformation campaigns and fraudulent activities.
Furthermore, it is surprisingly easy to expose sensitive information through the direct use of common generative AI utilities or inadvertently through poorly configured access controls. Sections A.4.b and B.13 of the Code of Ethics stipulate that planners must safeguard sensitive information about constituents and organizations, maintaining strict confidentiality unless disclosure is legally required. Planners must also adhere to organizational policies regarding the appropriate use of generative AI, exercising informed and independent professional judgment in its application.
Example: Protecting Sensitive Information
Alex, a planning consultant, is working on a housing needs assessment for a mid-sized city. The city has provided Alex with access to a database containing detailed household information, including names, addresses, income levels, and housing conditions. To expedite the analysis, Alex considers using an AI tool to process and summarize the data.
Before proceeding with the AI-assisted analysis, Alex can:
- Reflect on the sensitive nature of the data they are about to share with an external party.
- Review the local government's policy on the use of Generative AI if one is available and review a proposed workflow with the project team to confirm alignment.
- Clean the data before uploading by:
- Removing all personally identifiable information (PII) from the data
- Anonymizing the data
- Creating artificial data
4. Request outputs that would enable Alex to run analysis locally on their machine without compromising sensitive information.
Conclusion
The fundamental features of ethical planning practice remain keeping the public interest front of mind when navigating the complexities of human settlement for current and future generations. The scope of this article is purely to explore possible intersections of generative AI technologies with the AICP Code of Ethics and Professional Practice so that planners can hold themselves and their colleagues accountable for how this technology is used as part of ethical practice.
However, the authors understand there are large ethical and philosophical considerations beyond those listed here. Some of these considerations are discussed in other APA publications, but they range from the degree to which these tools disrupt public forums or enable harassment, the sizable environmental consequences of their training and deployment, and subtle concerns relating to 'value-lock' from models trained on static data. This is all to say, this is a deeply complex topic, but it is not the last word on it. More can be done to identify guidelines for privacy, data security, transparency, and fairness of applied AI in the planning process.
Additional APA Resources
For further interrogation of AI and planning ethics, please refer to other APA Resources. We recommend "The Ethical Concerns of Artificial Intelligence in Urban Planning," published in 2024 in the Journal of the American Planning Association.
- "Augmented: Planners in an Era of Generative AI," APA blog
- Artificial Intelligence and Planning, Research KnowledgeBase Collection
External Resources
- Generative AI Strategies, Planning Webcast Series
- AI Governance Alliance, World Economic Forum
Top image: iStock/Getty Images Plus - Natakorn Ruangrit