Big Data and Planning
PAS Report 585
By Kevin Desouza, Kendra Smith
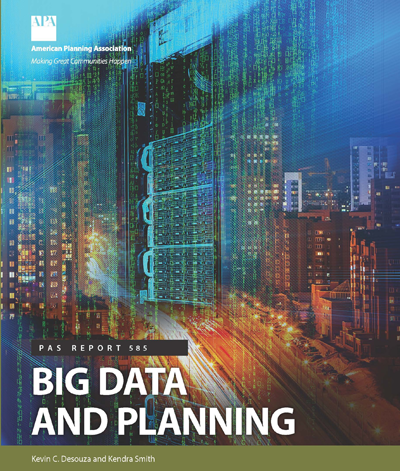
Not a member but want to buy a copy? You'll need to create a free My APA account to purchase.
Create account
This is big. Data sets are growing so large and complex that using them is like drinking from a fire hose. Feeling overwhelmed? Help is on the way.
Big data isn't the problem; it's the solution — and this PAS Report shows how to use it. Arizona State University researchers Kevin C. Desouza and Kendra L. Smith have teamed up on a practical guide to channeling the power of big data. Together they look at how planners around the world are turning big data into real answers for smart cities.
Learn how Dublin is gearing up geospatial data to steer traffic. See how Singapore is collecting citizens' selfies to track smog. Discover how Detroit is crowdsourcing creative ideas for its 50-year plan. And find out how the U.S. government is planning to use Yelp to improve its services.
What's the big idea for your community? Read Big Data and Planning for a look at trends and tools you can tap into today.
Executive Summary
We have more data than ever before on all kinds of things planners care about, ranging from fixed objects, such as buildings, to dynamic activities, such as driving behavior, and at all levels of granularity, from cities to individuals and everything in between. In addition, we have unprecedented access to data in real time, which gives us new capacities to monitor and evaluate the state of objects (artifacts) and agents. We have technology to thank for a great deal of this. Much of what we interact with is fitted with technology sensors, and our own (consumer) technologies — such as mobile phones, tablets, and fitness trackers — are also valuable sensors and emitters of data. Technology is not only generating these data but is also providing us with the computational tools to analyze, visualize, interpret, and act on large amounts of data. In addition, we have tools that are automating decision making through the embedding of intelligence, which is derived through analyses of large amounts of data.
PLANNING IN THE ERA OF BIG DATA
Digitization of all aspects of the city — from sensors embedded in our infrastructure to activity and movement tracking capabilities through GPS and even social sensing (crowdsourced data from sensors closely attached to humans, such as mobile phones or wearables) — allows us to learn about the sentiments of residents regarding policy and social options and provides a distinct opportunity to rethink how we plan and manage cities and communities. Key to this is the ability to leverage data through analytics in an effective and efficient manner.
Real-time analytics applied to data are also increasing our situational awareness about our environments. This increased real-time situational awareness will enable individuals, communities, and organizations to make more intelligent, or smarter, decisions. And, with smart technologies, there are greater opportunities to become aware of early warning signals through real-time access to sensors and predictive models that alert us to problems. The use of data, analytics, and smart technologies will help cities manage their complexities and become less reactive to challenges and threats.
Planners use various types of data and data sources in their complex plan-making activities. Big data has the strong potential to enrich various stages of plan making, including visioning, problem assessment, scenario planning, and plan implementation. Big data empowers planners and decision makers by helping them to better understand current situations and predict future ones more precisely and accurately. The intense amounts of time and labor required for data collection as part of comprehensive planning might be resolved in the near future with big data generated using volunteer sources or created through more formal processes. However, planners will need to be prepared to deal not only with data quality issues but also the institutional challenges of collecting, analyzing, and incorporating big data into planning processes.
WHAT ARE BIG DATA AND ANALYTICS?
A set of data is big when it is too large and too complex to be stored, transferred, shared, curated, queried, and analyzed by traditional processing applications. There is no specific size that is assigned to big data, as it is always growing. A more complex and accurate answer requires the understanding that data can be big in several dimensions. The simplest dimension is volume. We are talking about data that ranges in size from terabytes (1000⁴) to petabytes (1000⁵), exabytes (1000⁶), and zettabytes (1000⁷). With advances in computation, what is considered big today will be small in the near future, and what is gigantic today will be medium-sized in the short term.
The velocity at which data are generated and collected has undergone major changes over the last few years. Today we generate data at an accelerated rate across a multitude of environments. The lag between when an activity is conducted and when it is recorded has, for most things, almost disappeared.
The variety of data streams, formats, and types of data that we have access to has also exploded. Today, we have to contend with data that come across multiple streams — from formal systems to informal platforms (e.g., social media) — and formats including text and audio, visual and aural, and even olfactory. These data streams emit data with high degrees of variability in structure, frequency, predictability, and other characteristics.
Analytics is key to harnessing the power of big data. It is the process of connecting, analyzing, transforming, modeling, and visualizing data to discover valuable and actionable information. Two key activities take place during analytics. First, data are summarized and integrated to reduce their volume and converted into higher-level metrics and indicators that enable individuals to process the data. Second, the information generated is situated within the appropriate contexts in order to make sense of the data and conclusions to be rendered.
THE INTERNET OF THINGS
Through smart data and Internet of Things (IoT) technologies, more growth will happen in areas involving variables that previously were difficult to measure. IoT is the interconnection of devices that "talk" to each other, including personal electronics, sensors, and networks. Wearables, or wearable technology, is an IoT technology made up of clothing and accessories with embedded sensors and software that can connect to other objects or devices without human intervention. These devices will monitor specific conditions, such as motion, vibration, and temperature. The promise of IoT technology is a revolutionized, fully connected world where the environment and people are connected by objects.
For urban planners, IoT opens up a whole new realm of possibilities. Using this information, city infrastructures can be reimagined, which will have impacts on social, political, and environmental policies. Connected cars will make driving safer and cut down on carbon emissions while public spaces can adapt and adjust to users' needs to create entertainment, educational opportunities, and interactive spaces for gathering, collaboration, fellowship, and further innovation. This will all be done through insights gleaned from the collection of big data and analytics, insights that will be used to connect all facets of daily life. This might seem far-fetched now, but imagine 20 years ago, when floppy disks were used to save computer work and people used cameras with actual film that had to be developed in order to share physically printed photos with other people.
ANALYTICAL APPROACHES
Critical to the ability to leverage big data are analytical techniques. Traditional analytical techniques have to scale to handle the volume of data involved when dealing with big data. In addition, analytical techniques must be able to integrate different types of data and operate across a wide assortment of systems and platforms.
Open-Source Tools
The cost of analytics can range from free to expensive. For those planners not looking to break the bank on a new set of computational tools, there are open-source tools that are available at no cost. Open-source tools have source code that is openly published for use or modification from the original design — free of charge.
Data Mining
Data mining helps us discover latent patterns and associations between variables in large datasets. There are a multitude of analytical techniques that can be applied to uncover information from databases, including association rules, decision trees, and classification models. From a process point of view, generic data mining works the following way: the dataset is split into two (the training set and the validation set). Models that are deemed good have good predictive capacity and also have explanatory power (i.e., how much variance in the dependent variable is explained by the variables in the model). Association rules (or association mining) is a data mining technique that is probably the most well-known and straightforward. With this technique, one can discover the presence of an element in a dataset as it relates to the co-occurrence of other elements.
Machine Learning
Analytical approaches use algorithms to iteratively learn from data and build (and update) models. Machine learning approaches normally take one of two learning approaches: supervised or unsupervised. In supervised learning, the model is developed by analyzing patterns using a set of inputs and outputs. The analytical tool continues to learn and the prediction accuracy of the model improves over time. In unsupervised learning, data elements are not labeled and we allow the algorithm to identify patterns among the various elements. The machine normally will have to identify the latent structure in the dataset through a clustering approach to identify groups and clusters. Just as with data mining, several analytical techniques can be used for both supervised and unsupervised learning depending on the size, structure, and format of the dataset.
Sentiment Analysis
Today, given the popularity of social media and the proliferation of commentary on these platforms, sentiment analysis (or opinion mining) has become a popular analytical approach. At its core, sentiment analysis leverages techniques in natural language processing, computational linguistics, and text analysis to uncover information from large bodies of text. Machine learning approaches that focus on sentiment analysis are often used when assessing polarity. Opinions — gathered from sources such as tweets and Facebook posts — are placed in a huge bucket of words that human coders have decided they would like to study. The algorithms not only work to classify words on various dimensions (e.g., positive/ negative, level of authenticity, confidence) but can also be used to uncover latent connections between words and collections of words (i.e., phrases).
Geographic Information Systems
A critical element of big data analysis is the layering of data elements to get richer contextual information on environments. From a spatial perspective, leveraging geographic information is critical. Geographic information systems (GIS) deliver various forms of information as multilayered maps in which each layer provides different information. These systems have evolved from complex and difficult applications to essential parts of how citizens and governments understand and relate to their environments. Increasingly, public agencies are using GIS to capture, store, analyze, and manipulate data to decrease costs, increase communication, and improve decision making. The advancements in visualization and information technologies have further accelerated the use of GIS in the public sector in fields such as emergency management and urban planning.
Network Analysis
The exploration of connections helps us understand how networks are structured and how they evolve. Network analysis allows us to see the connections among various data elements. For example, we can see the connections between two agents (humans) or two objects (devices) as well as those that occur between humans and objects (e.g., two humans who are connected to or dependent on the same object — say, an energy source). Today, urban planners must become comfortable dealing with large datasets where computation of networks is not as straightforward as when only a single form of interaction or connection was considered. One of the attributes of big data is the ability to link datasets (i.e., connect data elements) across various domains.
Agent-Based Modeling
In agent-based modeling (ABM), computational models of complex systems are developed by specifying the behavior of agents (individuals or organizations) and their resources, behaviors, preferences, and interactions as well as activity with other agents, objects (artifacts such as buildings, systems, and land), and the environment. Each agent corresponds to a real-world actor who is cognitively and socially bound to a place. The simulation allows one to trace how collective patterns emerge from the interactions between agents and their environments given the rules and constraints modeled. The interaction rules, constraints, and environmental conditions are modeled to represent the options being considered.
Gaming
Planners can also use big data to capture activity, interest, and interaction through gaming. Gaming is a useful and fun method of experimenting and making decisions through gamification, or using game elements in nongame contexts. The point of gaming is to always understand the behaviors of users and what motivates these behaviors. Gaming is a benefit to urban planning because it takes user activity, captured through big data, to understand interactions and decisions. Data collected from games can aggregate such issues as common problems that arise, people's most immediate decisions, what motivates behaviors (e.g., instant gratification, incentives), and what was learned on subsequent tries of the game. Further, gaming can also help transform big data into smart data. This happens through turning user decisions into data-driven insights.
Building Information Modeling
Building information modeling (BIM) is a process of digitally representing the physical and functional characteristics of places such as buildings, tunnels, wastewater and electrical facilities, ports, prisons, and warehouses. BIM is a departure from the 2-D technical drawings that are used in traditional building design. It extends beyond 3-D to a fourth dimension of time and a fifth dimension of cost. BIM provides opportunities to use IoT and other tools, such as GIS, to combine and analyze physical and administrative data (such as vacancies and lease space) and other data sources like LIDAR laser information.
A FRAMEWORK FOR LEVERAGING BIG DATA THROUGH ANALYTICS
The analytical process needed to use big data requires rigor and attention to detail. This process needs to ensure that data are collected from the most appropriate sources, validated, and integrated. In order to do this, appropriate analytical techniques need to be employed, analytical outputs should be carefully scrutinized, and relevant insights and actions should be implemented and disseminated in an optimal manner. The following four-phase framework can help guide big data users through the process.
Phase 1: Management of Data Sources
Data are generated by agents (i.e., humans) and objects (i.e., things). Traditionally, planners were confined to a limited number of sources from which they could collect data. These sources produced data on a regular basis and in defined forms, and they were deemed credible due to their official designations, such as the U.S. Census Bureau, or the regulated processes they followed. Today, things are quite different. In addition to traditional sources, planners have available data from a much wider variety of sources. Many of these sources do not have the same credibility or authority as traditional data sources. They also often lack permanency and emit or produce data that are of varied types, frequencies, and formats. Source management is about knowing what sources one should pay attention to, being able to evaluate the credibility and veracity of sources, extracting data from these sources when needed, organizing sources, and protecting sources and the data being extracted.
Phase 2: Information through Analytics
The next phase starts the analysis process that allows for the extraction of information. When data scientists and analysts receive data, the data will often be in formats that are incorrect, inconsistent, inaccurate, irrelevant, or incomplete. This requires cleaning and correcting of the so-called "dirty data." Data cleaning is not optional, and this task is critical to the analytical process. Without it, insights derived from the data can be incorrect and misguide planning and public policy actions, such as financial or resource allocations or improvements to infrastructure.
Once data from several sources are cleaned, the next step is to link and connect them so as to bring multidimensional perspectives and broaden one's situational awareness. The fusing of data is a nontrivial task that requires creativity and rigor. Creativity is important because identifying the fields that can be linked or connected in a database is not always easy to do, and hence a fair degree of innovation takes place. This is especially true when trying to link databases that do not use the same fields consistently from one database to another. Rigor is important to ensure that the data are not incorrectly manipulated or accidentally transformed during the integration.
A wide assortment of approaches is available to analyze data; several of the common approaches were discussed earlier. While data scientists can conduct analyses, analytic expertise can come from other sources, including community collaborations such as hackathons and crowdsourcing platforms. More traditional analytical tools are available (e.g., software such as SAS and SPSS). Another source are the thriving open-source communities building analytical tools. These tools are made available to the public in the form of source code, technical manuals, and user communities that work on next iterations of these solutions, share feedback, and fix and report bugs.
Phase 3: Interpretation of Data Outputs
This phase is all about ensuring that analytical results are being interpreted in the most accurate manner and within the appropriate context. Analytical outputs can easily be misinterpreted both accidentally and purposefully to advance an agenda. It is important that care be taken so as to not fall into common traps when interpreting analytical outputs. First, interpretation of results in a timely manner is essential. Insights that are outdated and no longer useful are a waste of time for all parties involved. Setting up organizational processes that make data interpretation a regular part of operations is essential to making timely interpretations. Second, the right people should be engaged in the interpretation process. These can be individuals internal to the organization, outside experts, and the public (usually through crowdsourcing activities).
The manner in which analytical outputs are shared is an important decision. Outputs can be shared via reports that describe the results, provide context, and examine the pros and cons of the recommendations or outcomes. Visualizations are a method of sharing analytical outputs using platforms that help create awareness and provide valuable insights into how a city is performing. There are many different visualization types that run the gamut from static visualizations, such as infographics, to dynamic and interactive ones, such as advanced heat maps.
After analytics have been shared, researchers should solicit feedback on the interpretations. This includes critical questions, suggestions, and ideas about future work. It is important to understand the implications of the knowledge in question before constructing a course of action. Feedback can be gathered from citizens, individuals internal to the organization, and outside experts. Citizen feedback can happen in town hall or community forums or through websites with comments sections or citizen engagement platforms.
Phase 4: Design and Implemention of Evidence-Driven Policy
Once the analytics are complete, the process of considering various policy options and designing strategies to implement outcomes begins. When possible, it is advisable to run experiments to test the efficacy of policy options and to study both the intended and unintended consequences of courses of action. Experimentation is a process that planners may not be comfortable with, but it is important to understand how you might go about implementing solutions. Implementation of a solution can take many forms, and often there are tradeoffs involved with each possible trajectory. Experimenting might allow you to see how well you witness the intended consequences and to identify some of the unintended consequences. Experimentation is risky, as it might reveal things that warrant going back to the drawing board and beginning again. However, experimentation always leads to learning, which is vital.
When outputs from analysis have been interpreted, shared, and tested through experimentation, they have become actionable insights and reached their potential. At that point, it is then in the hands of decision makers to drive new, evidence-based policies and processes. Policy makers and researchers have recognized that evidence-based policy making based on rigorous evidence is a better way to operate efficiently and strategically. The availability of evidence (using big data and analytics) can help planners understand what is working and what is not.
An interesting option with big data and analytics is to continuously evaluate policies to ensure that the desired implementation and impacts are achieved. It is vital that agencies develop formal processes to evaluate policies on a regular basis. There are several reasons for this. First, conditions change regularly. Any policy that is deployed will need to be revised as conditions in a community change. Second, feedback collected from the evaluation of policies provides opportunities to make small modifications and tweaks. This is much more advisable then waiting until it is necessary to overhaul the entire infrastructure and system.
THE FUTURE OF BIG DATA AND PLANNING
The future of analytics and big data is bright and will transform the practice of planning. While it is always challenging to predict with pinpoint precision how things might play out, there are several trends that warrant attention. First, there will be a growing movement to integrate traditionally disparate databases at the city, state, and regional levels. Today, gaps exist that prevent us from getting a more holistic view of how individuals engage with public services and agencies. Simply put, layering of multiple datasets allows deeper and more precise insights into phenomena. Second, the trend to combine analytics with know-how in terms of behavioral sciences is picking up momentum. Third, the trend of the automation of almost everything is in full swing. Automation will also affect how data are collected and who analyzes the data. Fourth, given how much people's lives, organizations, and societies have come to depend on the digital information infrastructure, it is not surprising that the unscrupulous want to disrupt and cause harm to these systems. In the future, municipalities and different government agencies will need to find ways to coexist; for instance, officials concerned with big data and other technologies must learn to function effectively with officials concerned with privacy and security. Fifth, the rise of the nontraditional planner and the growth of crowdsourcing platforms mean that we are likely to see many more solutions developed outside of local government.
Urban planners play an important role in most cities as they create and implement visions for communities. They lead efforts around social change, policy making, community empowerment, sustainable growth, innovation, disaster preparedness, economic development, and resilience. Planners also solve problems through research, development, and design. Fundamental to carrying out these activities are their abilities to make decisions in effective and efficient manners. To increase their decision-making capacities, it is critical for planners to leverage data in innovative ways. The future offers exciting possibilities, and planners are encouraged to stay abreast of developments in data science and to find ways to begin conversations with city leadership about how these technology-based opportunities can advance their communities.
About the Authors
Kevin C. Desouza, PhD, is a foundation professor in the School of Public Affairs at Arizona State University (ASU). For four years, he served as associate dean for research for the College of Public Service and Community Solutions. He is also a nonresident senior fellow at the Brookings Institution. Immediately prior to joining ASU, he directed the Metropolitan Institute in the College of Architecture and Urban Studies and served as an associate professor at the School of Public and International Affairs at Virginia Tech. From 2005 to 2011, he was on the faculty of the University of Washington (UW) Information School and held adjunct appointments in the College of Engineering and in the Daniel J. Evans School of Public Policy and Governance. At UW, he co-founded and directed the Institute for Innovation in Information Management (I3M); founded the Institute for National Security Education and Research, an interdisciplinary, university-wide initiative in August 2006 and served as its director until February 2008; and was an affiliate faculty member of the Center for American Politics and Public Policy.
Kendra L. Smith, PhD, serves as a policy analyst at the Morrison Institute for Public Policy at Arizona State University (ASU). Previously, she served as a post-doctoral scholar in the College of Public Service & Community Solutions and research fellow in the Center of Urban Innovation at ASU. She is interested in a wide range of public sector issues, such as innovation, citizen engagement, urban planning, and technology adoption, as well as how the public and private sectors can develop mutually beneficial partnerships to enhance innovation, efficiency, and effectiveness for society. She understands the need for utility and real-world applications for public sector research and seeks to place solutions directly in the hands of public administrators through publications, consultation, and advisement. Her work has been featured in Stanford Social Innovation Review, Governing, and Public Management (PM) as well as on NPR and through the Brookings Institution.
Product Details
Table of Contents
Executive Summary
Chapter 1. Introduction
Planning in the Era of Big Data
Appreciating the Complexity in Planning
This Report
Chapter 2. Big Data and Analytics: The Basics
What Is Big Data?
What Is Analytics?
The Internet of Things: Opportunities for More Data
Going Forward
Chapter 3. An Overview of Analytical Approaches
Tapping into Open-Source Tools
Data Mining
Machine Learning
Sentiment Analysis
Geographic Information Systems
Network Analysis
Agent-Based Modeling
Gaming
Building Information Modeling
Conclusion
Chapter 4. A Framework for Leveraging Big Data Through Analytics
Phase 1: Management of Data Sources
Phase 2: Information through Analytics
Phase 3: Interpretation of Analytical Outputs
Phase 4: Design and Implementation of Evidence-Driven Policy
Conclusion
Chapter 5. The Future of Big Data and Planning
References
Acknowledgments